The Triple Win of Centralized Statistical Monitoring
entralized statistical monitoring (CSM) can identify data quality issues at least three times faster than traditional approaches, saving sponsors hundreds of thousands of dollars and providing robust, credible results.
The project was designed to evaluate the ability of a centralized monitoring and analytics platform to effectively identify sites with known quality issues on a previously completed clinical study. The team had been tasked with identifying problems in site data that the sponsor had only discovered late in the study’s execution phase, and demonstrate how early CSM would have flagged the issue.
The second part of the challenge was of particular importance. The objective of centralized monitoring is not simply the ability to detect operational risks, but to detect their emergence early enough that corrective actions can be taken to limit potential damage to subject safety and protect the credibility of study results.
Background: Taking the Risk-Based Approach
The approach, endorsed by both FDA and EMA and encoded in the ICH E6 (R2) GCP update, encourages less reliance on traditional on-site monitoring practices, such as frequent site visits and 100% source data verification/review (SDV/SDR), in favor of centralized monitoring. A key part of this is using statistical methods to identify quality-related risks such as unusual or unexpected data patterns during the study.
Over the last few years, a growing body of evidence has emerged supporting CSM over the relatively low value of comprehensive SDV and SDR. Such evidence includes an analysis conducted in 2014 showing that the traditional practice of 100% SDV (which comes at a very high cost) results in corrections to only one percent of the data on average. However, many organizations have struggled to fully understand and appreciate the power of this approach, which has been shown to drive higher-quality outcomes and allow sponsors to direct precious resources to sites that need attention.
The quality data challenge aimed to shed light on the practicalities and tangible benefits of embracing this “new way” of doing things.
Case Study: Data Quality Challenge
The project was divided into two phases (Figure 1). The objective of the first was to confirm if CSM data review would identify the site issues; the second to find out how early such a review would be able to flag the problems.
While the quality issues were known to the clinical trial team, the people involved in the data quality challenge remained blinded until after the analysis was complete and findings presented.
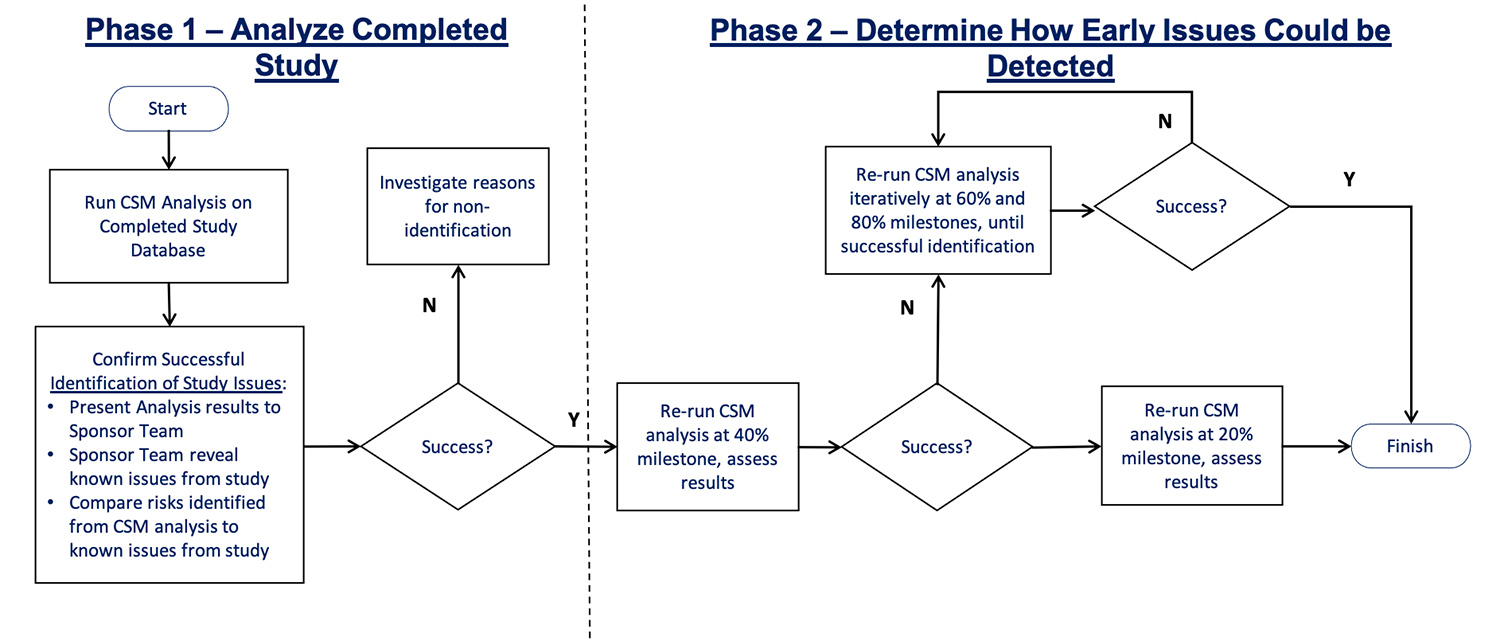
Unearthing Problem Data: Phase 1
- Data quality assessment (DQA) by site: DQA is an advanced set of statistical tests implemented across the full suite of study data to identify unusual patterns at sites, regions, or among patient groups.
- Key Risk Indicators (KRI) by site: Any number of standard and study-specific KRIs can be configured to help identify specific risks of interest across sites, regions, or among groups of patients. For this project, eight KRIs were selected and designed based on a review of the study protocol.
Other modules also configured for the data quality challenge were duplicate patients’ identification, patient profile reports, and quality tolerance limits (QTLs).
The DQA statistical tests generated hundreds of p-values per site, a weighted average of which was computed and converted into an overall data inconsistency score (DIS). Four sites with the highest DIS were flagged as “at risk,” and their risk signals and KRIs were then reviewed by the team.
Site 104
The study team reported that while no significant issues had surfaced during the conduct of the trial, concerns had been raised during the primary analysis of the completed study database. All enrolled patients at this site had anomalous data patterns for creatinine clearance rates (eGFR), the study’s primary endpoint measurement.
The site, which had enrolled the second highest number of participants across the whole study, had recorded improbably high values and significant variations in eGFR. It also had a suspiciously low adverse event (AE) reporting rate. Two months after study completion, the site was submitted to an audit. All of the site’s data was deemed unreliable and excluded from the primary analysis.
It’s worth noting that CSM statistical monitoring not only clearly identified the primary issues observed by the study team (i.e., the anomalous eGFR results and very low AE reporting) but it also found highly suspicious patterns of data in the vital signs and various other central lab results reported at site 104.
What Might Have Been: Phase 2
In the second phase of the data quality challenge, the team re-ran the analyses iteratively on earlier versions of the database. At both 40% and 20% of progress milestones, they once again successfully identified site 104 as an at-risk site.
In fact, anomalous patterns of eGFR data, very low AE rates, very low variability in blood pressure measurements, and anomalous patterns of data in several additional central lab results, were all flagged at the 20% progress milestone. This equates to eight months after initiation of the study, compared to the 26 months that was needed using only traditional data review methods.
Initial risk identification by month eight could have led to an investigation and audit by month nine, resulting in remediation at least 16 months earlier than what actually transpired. The benefits of this for sponsors are significant. The reduction in study costs, including payments to the site and management costs beyond month 10, are estimated at $351,100 alone (Figure 2).
Cost Driver
Months 10 to 23
Cost Driver
Months 10 to 23
Cost Driver
Months 10 to 23
Cost Driver
Months 10 to 23
With CSM, the study team could have identified the problem at the 20% progress milestone, carried out an audit, and then made up for the loss of fifteen patients through recruitment the other sites. However, this option was not available, leaving the study team with the possibility of significantly reduced statistical power in the endpoint analyses. Ultimately, this might have jeopardized the planned marketing approval submission.
In this case, endpoint sensitivity analysis concluded that no power was lost in the endpoint analysis. But there’s no avoiding the fact that the potential business implications of this scenario could have been both severe and costly.
Future of CSM
This fundamental difference means that CSM operates at a higher, more contextual level, making it more effective at identifying site and study issues that are likely to be significant.
This does not mean that well-placed data management reviews and automated EDC queries no longer play a role. They still add value to the quality oversight process. They are relatively low-cost activities with a meaningful return on investment and they help to remove the reliance on comprehensive SDV and SDR as a primary monitoring activity.
But this case study adds to the growing body of evidence that effective CSM is a powerful way to proactively identify emerging operational risks during the conduct of clinical research, and also demonstrates why ICH E6 (R2) guidance states that CSM, along with well-placed KRIs and QTLs, should be considered an essential component of any centralized monitoring implementation. Furthermore, it provides a solid understanding of the tremendous, tangible value CSM can offer in achieving the ultimate triple aim of drug development: lower direct costs, shorter timelines, and more successful study outcomes.