Part 1: R&D Applications and Approaches
AstraZeneca
CGI
his article is first in a series that explores the potential of AI in the healthcare sector and paves the way to uniting the global community of patients, data scientists, medical professionals, and clinical representatives. Subsequent articles in future issues of Global Forum will examine specific use cases within healthcare, the regulatory landscape surrounding AI implementation, the integration of human-centric AI, change management strategies, and optimizing synergy between artificial and human intelligences.
Understanding AI: Unraveling Layers of Technological Ingenuity
AI encompasses a spectrum of technologies, each contributing distinct capabilities to the evolving landscape of pharmaceutical innovation. AI is a broad area, with machine learning (ML) and deep learning (DL) at the top. Figure 1 illustrates the cascading layers from generic concepts to specific technology applications, moving from Generative AI (GenAI) to large language models (LLM), Generative Pre-trained Transformers (GPT), GPT-4, and finally ChatGPT. ML empowers systems to learn from data, recognize patterns, and make informed decisions without explicit programming. DL, a subset of ML, mimics the intricate architecture of the human brain by representing neurons as mathematical functions which handle language, speech, images, and videos.
As we move through these layers of AI sophistication to cross the traditional predictive or discriminative AI that deals with existing data, GenAI welcomes a new paradigm where machines transcend analysis and venture into creativity, capable of autonomously generating new, meaningful content. The potential of GenAI holds immense promise in the context of drug development by offering innovative solutions to complex challenges and reshaping the trajectory of pharmaceutical discovery.
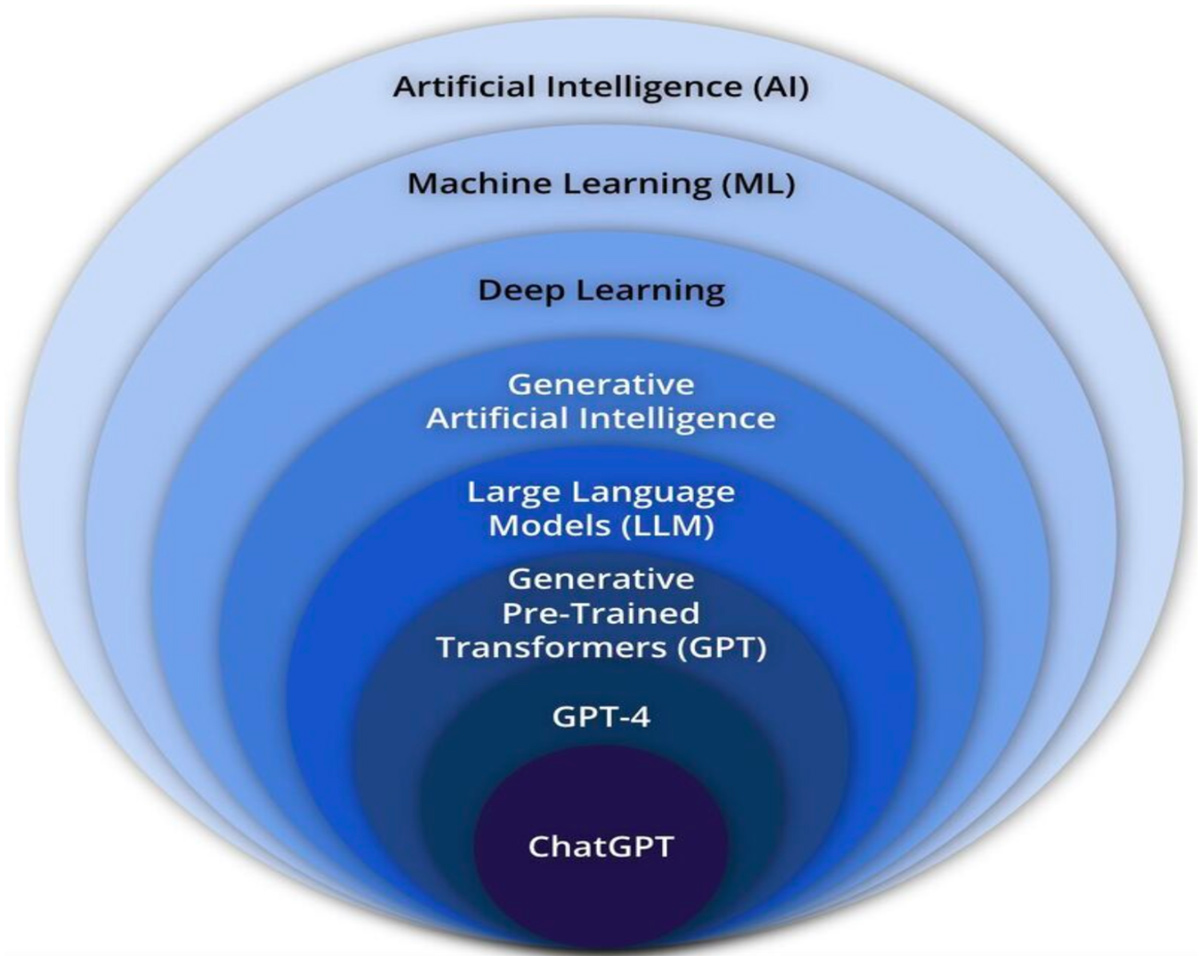
Deep Dive into Generative AI
Akin to an artist’s palette of colors, generative AI task capabilities can be amalgamated to fulfill the demands of specific use cases. Just as artists blend colors to craft their masterpieces, these capabilities blend to generate diverse outcomes.
The foundation of LLM is natural language processing (NLP), which is about understanding and processing human language. LLMs build upon this; GenAI is the leap from understanding and processing to creating. These NLP options are increasingly permeating everyday applications within every functional area. Some key applicable tasks pertinent to drug development and healthcare include:
Semantic Search: The interplay between “tacit knowledge” and “explicit knowledge” is the major driver in knowledge management and its outcome: innovation. Digital assets (explicit knowledge) and the human experience (knowledge worker) are the seeds of idea generation, problem solving, and value creation within an organization. Traditional semantic search (pre-LLMs) used techniques that went beyond simple keyword searches—for example, keyword expansion, latent semantic analysis (LSA), vector space models, etc.—but was limited. Semantic search enhanced with generative AI takes this capability to the next level because it possesses deep contextual and semantic understanding of both the user query AND the organizational-explicit knowledge assets. This is the game changer: efficiently mapping these two together creates disruptive change. In healthcare, there are many areas where this enhanced capability can add value to “low-hanging fruit.” Three simple examples:
Improved Search Engines: Enhancing traditional search engines to better understand user queries and deliver results that match the searcher’s intent and context, not just keywords. One example is searching information management systems to generate insights.
Customer Support: Automating and improving customer support through chatbots and virtual assistants that understand customer queries in a more humanlike manner, providing more accurate and helpful responses. “Customer support” can also serve internal (organizational) stakeholders.
Decision-Making: Supporting clinical decision-making by understanding and retrieving relevant medical literature and patient information based on complex search queries that involve symptoms, diagnoses, and treatments, and account for the context and specifics of each case.
In addition, after finding explicit assets via semantic search, generative AI can synthesize data through text generation, another generative AI capability, to produce easily digestible outputs for the user. Google Search Engine is currently offering this to its US user base; this experience can be expanded within an organization using organizational content.
Figure 2 below illustrates several applications of Generative AI useful in pharmaceutical or life sciences R&D:
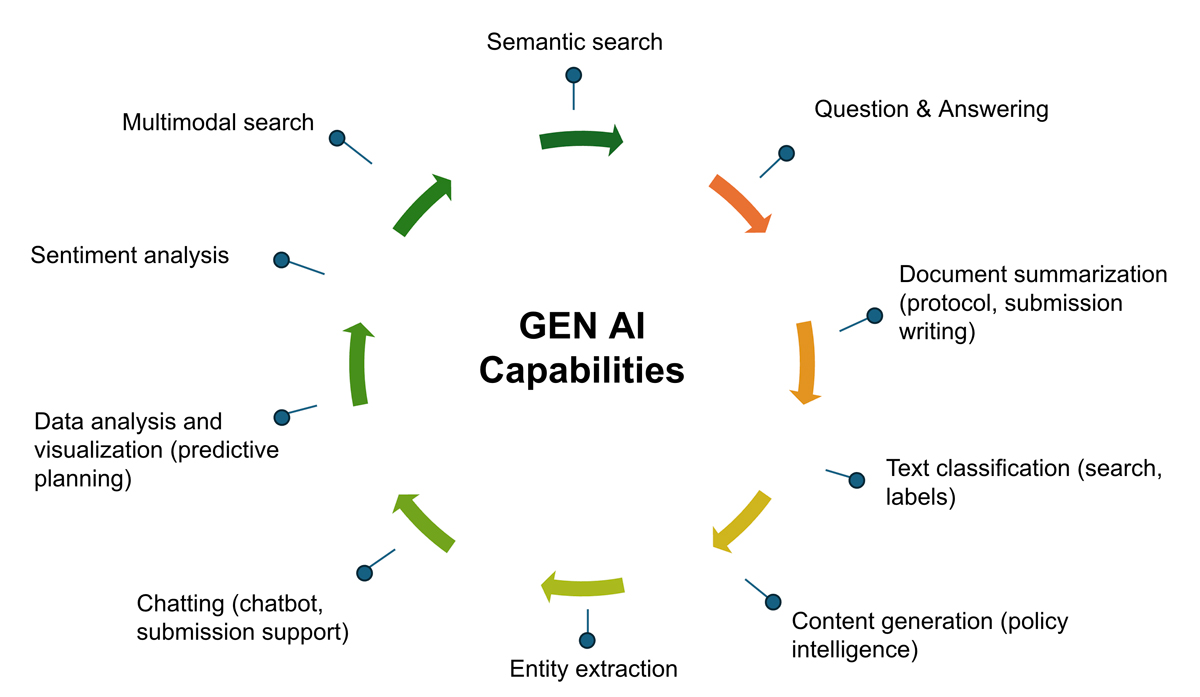
Document Summarization: One best practice that creates value in good documentation is to include a summary and key points of interest along with the original content. In today’s information overload, this key capability expedites interaction between explicit knowledge and human knowledge. There are many methodologies and approaches for leveraging LLM models for document summaries and, depending on the use case, may include extractive summarization, abstractive summarization, hybrid summarization, fine-tuning, and multidocument summarization. (See accompanying sidebox.)
Abstractive Summarization: Unlike extractive summarization, abstractive methods generate new phrases or sentences that are not necessarily found in the source document. LLMs can be trained to understand the context and meaning of a document and then articulate a concise version of it in new words, often more succinctly and fluidly, imitating a human’s ability to summarize content.
Hybrid Summarization: This approach combines both extractive and abstractive techniques. An LLM might first identify key sentences or segments using extractive methods and then rephrase or generate new sentences that blend these extracts into a coherent, abridged version of the original document, leveraging the strengths of both approaches.
Fine-tuning with Specific Data: For domain-specific document summarization (e.g., regulatory, medical, or technical documents), LLMs can be fine-tuned with targeted data sets. This process involves training the model further from a corpus of specific examples to enhance its understanding and summarization capabilities within that domain.
Multidocument Summarization: This approach involves summarizing information from multiple documents into a single cohesive summary. LLMs can be trained to identify and synthesize overlapping, complementary, or contradictory information across various sources, producing a summary that captures a broader perspective on a topic.
Text Classification and Tagging: Content in knowledge management systems, especially compliance documentation, requires consistent tagging. Knowledge does not deliver full value if only the author and their immediate team can find it and put it to use. Metadata is also important for requirements such as filtering and dashboard reporting and is essential during content migrations.
LLMs can automate metadata tagging and present tags to humans for confirmation or tag the content without humans in the loop if confidence in the LLM is high enough. AI-powered tagging improves the usability of organizational knowledge assets. Text classification models can tag adverse events mentioned in clinical trial reports or patient narratives to MeDRA or SNOMED CT, helping researchers to analyze the reports rapidly.
Entity Extraction: The ability to read a document and extract key elements such as locations, diseases, study sites, equipment, and/or people, and use them for subsequent processing such as metadata identification, knowledge graphs, and searching, is a key requirement in drug development. LLMs offer a great advantage over traditional metadata extraction methods because they mirror human behavior and interpretation instead of conducting simple keyword or rule-based searches (which are complex to establish and maintain). LLMs can perform Named-Entity Recognition (NER) to identify and classify entities within medical text. For example, sentences like The 45-year-old woman presented with symptoms of hypertension and diabetes and underwent a CT and showed signs of MI would be extracted for clinical concepts or medical terms from text, capturing the meaning and context of these terms like patient demographics, disease names, and CT (computed tomography) and MI (myocardial infarction).
Chatbots: Chatbots powered by generative AI models simulate humanlike conversations to provide users with assistance or retrieve information through text or voice interactions. This synergy is paving the way for more intuitive and humanlike digital interactions within the workplace, converting the knowledge worker’s output to more value-adding outcomes. Some very powerful applications of chatbots include enhancing the patient experience by providing responses to common patient questions or using chatbots in the supply chain for planning, procurement, or payment processing.
Data Analysis and Visualization: GenAI is making significant strides in data analysis and visualization by automating and enhancing processes involved in interpreting and presenting complex data sets. By learning from vast amounts of data, generative AI algorithms can identify patterns, trends, and correlations that might not be immediately obvious to human analysts. This capability allows for generation of insightful, detailed visual representations of data such as dynamic graphs, heat maps, and interactive simulations tailored to users’ specific needs and questions. When dealing with regulatory submissions across the globe, it will greatly help pharmaceutical companies to have a real-time dashboard of submission activities. GenAI can also simulate various scenarios based on historical submissions data and provide predictive analytics that are visually represented to aid decision-makers in exploring future possibilities and outcomes. This integration of generative AI with data analytics and visualization tools accelerates the data-to-insight pipeline and democratizes access to advanced analytics.
Sentiment Analysis: Generative AI is revolutionizing sentiment analysis by enhancing the ability to understand and interpret the nuances of human emotions from textual data. Using AI in RWE offers a multifaceted understanding of patient sentiments beyond traditional quantitative metrics. Generative AI models can leverage vast data sets to discern subtle variations in tone, context, or language usage that indicate sentiment, whether positive, negative, or neutral (or any other sentiment mix that the use case and training data determines). This advanced capability enables more accurate and detailed analysis of customer feedback, social media interactions, and any textual communication, providing insights into people perception and emotional responses.
Moreover, generative AI’s adaptability enables it to continually refine its analyses as it learns from new data inputs, enhancing the accuracy and relevance of sentiment assessments over time. This iterative learning process empowers healthcare stakeholders to stay attuned to evolving patient perceptions and preferences, facilitating informed decision-making across various facets of healthcare delivery and product development.
By leveraging generative AI for sentiment analysis in RWE, for example, healthcare practitioners, pharmaceutical companies, and policymakers gain invaluable insights into patient satisfaction, treatment efficacy, adverse events, and other crucial factors shaping healthcare outcomes.
Multimodal Search: Generative AI is significantly advancing multimodal search by creating systems capable of understanding and processing queries across multiple types of data inputs—text, images, audio, and video—to deliver more accurate and relevant search results. For radiologists or analysts accessing data across hospital systems, this allows for a richer, more intuitive search experience where users can, for example, upload an image and receive related textual information or vice versa. The evolution towards multimodal search not only enhances the user experience by making information retrieval more flexible and powerful but also opens new possibilities for how healthcare professionals interact with and leverage digital content across various domains.
The above is just a sample of what is available and is already adding disruptive value in other industries. Subsequent articles will examine use cases, governance models, innovation models, practical recommendations, the regulatory landscape, and related topics.
Building a Collaborative Community
In order to include every stakeholder and realize the full potential of AI in the life sciences and healthcare industries, it is essential to establish and leverage a collaborative community where data scientists, medical professionals, and industry experts come together to openly discuss, share their expertise and insights, and learn from others. By bringing together stakeholders with diverse expertise and perspectives, collaboration enables the development of ethical, effective, and trustworthy AI solutions that ultimately benefit patients, healthcare providers, and society. There is a plethora of information on the technical aspects of AI at the developer level, but spaces to collaborate on the “how” are lacking. We seek to address this gap.
Collaborating ensures that AI solutions are developed, deployed, and utilized in a manner that aligns with the diverse needs and requirements of these stakeholders. Cooperation allows for the integration of clinical insights, ethical considerations, regulatory compliance, and patient preferences into the design and implementation process, resulting in more robust and effective solutions. In the past decade, AI has undergone rapid evolution, with breakthroughs in various domains. One of the most notable recent developments in the realm of AI is the release of ChatGPT, a conversational AI model developed by OpenAI. The success of models like ChatGPT can be attributed, in part, to the collaborative and open nature of the AI community. Researchers and practitioners around the world freely share their findings, code implementations, and data sets, fostering a culture of knowledge exchange and collective learning. Open-source initiatives play a crucial role in democratizing AI research and enabling rapid innovation by lowering barriers to entry and facilitating collaboration among diverse stakeholders.
This article also serves as our invitation to stakeholders across the healthcare spectrum to join DIA’s AI in Healthcare community and contribute their knowledge, experience, and perspectives to this seismic transformation. By building this collaborative framework, we seek to cultivate an environment where innovation thrives and the transformative potential of AI is harnessed for the betterment of global health, creating a future where cutting-edge technology and collaboration converge to redefine drug discovery and development and tackle increasingly complex challenges to unlock new opportunities for innovation and discovery.
This article is the first in a series; look for subsequent articles in upcoming issues of Global Forum.