Part 1: The Intersection of Analytics and Quality Management
AstraZeneca, Canada
AstraZeneca, UK
here is a clear conceptual link between Quality Management and Analytics, encapsulated in the maxim “You can’t manage what you can’t measure.” In practice, however, many pharmaceutical organizations struggle to operationalize this idea: Quantifying quality is filled with challenges, both technical and organizational, including a lack of essential data, siloed teams, decentralized systems, and lack of a unified roadmap for uplifting quality reporting and analytics.
What is Analytics?
Analytics refers to the systematic analysis of data. It involves discovering meaningful patterns, correlations, and trends within data sets to derive insights used to make informed decisions and predictions. In a business context, analytics is used to optimize performance, improve efficiency, and solve complex organizational problems. Broad application of this definition of analytics encompasses a spectrum of capability including descriptive reporting, diagnostic analysis, and predictive analytics (see figure 1). There are higher tiers beyond this—for example, prescriptive analytics—but this article addresses only these first three tiers.
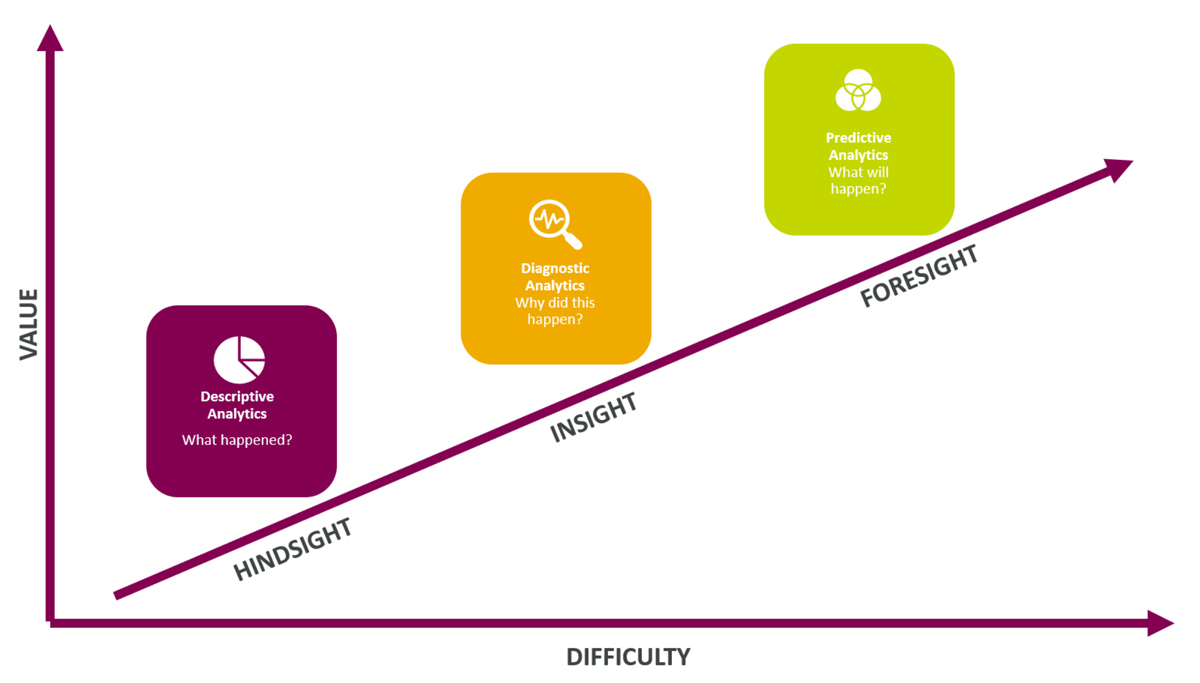
Making Quality Measurable: Embedding Analytics
Embedding analytics within organizations generally, and within quality functions, is a process that occurs in stages. These stages roughly correspond to the three descriptive, diagnostic, and predictive tiers mentioned above: Organizations start by trying to establish the basic facts; then to understand them; and then to use this understanding to predict the future. This section will share three case studies as practical QMS illustrations of each tier of analytics capability. First, more detail about these tiers:
The goal of descriptive reporting is to integrate systems and establish a centralized source of truth for consistently communicating basic facts. In a quality context, this might be as simple as reporting the number of Quality Events (QEs) that have occurred in the last quarter but will generally be more specific and tied to a KPI, e.g., the percentage of QEs that have closed on time.
After this bedrock is established, the next step is to move beyond the basic facts by understanding what is driving them. For example, suppose that an organization has noticed a decline in the percentage of QEs closed on time and wants to understand whether the decline is being driven by a particular cause or causes. In organizations without diagnostic reporting, this will likely involve assigning an analyst or business contact to provide a human interpretation based on reading the individual event records line by line, looking at their categorization, textual descriptions, etc., and writing a report. However, once diagnostic reporting is in place, the inconsistency and bias present in the manual approach can be removed by extracting the correlations, identifying seasonal trends, and so on, to isolate the underlying relationships.
The move to diagnostic reporting builds and is dependent upon the descriptive tier; diagnosis is only possible where a consistent factual bedrock exists. The same is true of the move from diagnostic to predictive reporting, where the relations in the underlying data are repurposed to look forward. Here, data science can add significant value to quality organizations by enabling them to predict, e.g., whether a certain site is likely to experience inspection.
With these divisions in mind, here are detailed examples of challenges and solutions at each tier.
Case Study #1: eTMF Document Status Reporting
Across study lifecycles, a persistent need for study teams is the ability to report on the timely creation and filing of essential documents within the electronic Trial Master File (eTMF). For example, teams need to ensure during start up that their data management documents are filed before the first subject enters the study. Similarly, teams need to ensure that all significant adverse events (SAEs) are reconciled before clinical data lock.
Having descriptive reporting ready is important since an incorrect or late filing, or missing documentation, results in a quality issue report as well as potential audit/inspection finding. Even worse: If these documents are missing or late without explanation during an inspection, a further finding may be raised.
A crucial step to ensure quality in the prompt production and submission of documents is to determine which documents have approaching deadlines and to alert the appropriate team members to prepare for their timely filing. In the absence of centralized analytics, this creates a significant burden for study teams. The challenge is that the standards which specify the timelines for different document classes are diverse and typically specified only in standard operating procedures (SOPs) as unstructured text, e.g., as a .doc(x) or .pdf file. (This document might specify, for example, that a Data Management Plan must be approved before the first patient enters the study.) This makes it difficult for a study manager to generate a simple system report which classifies relevant documents according to their delivery status: They can generate a list of documents filed, but this doesn’t tell them whether the documents were filed in accordance with the SOP, much less whether any are missing or coming due. As a result, study teams will often spend considerable time and effort exporting raw data into spreadsheets, running supplementary reports to determine the relevant milestone dates, and then manually comparing them through reference to the SOP document.
One company automated the classification of document statuses and developed a reporting suite that enabled study teams to rapidly understand the state of their study plans. The key technical requirement was translating the unstructured data contained in diverse SOPs into structured data with clearly defined fields and categories suitable for analysis and reporting. A system table stored the relevant data for each SOP (see figure 2). (This is a simplified example: In production, a system table such as this would use internal identification numbers for milestone and document classes rather than their labels and would likely require additional specifications. For example, many SOPs specify that specific document versions must be accounted for.)
This very simple technical innovation demonstrates the type of low-hanging fruit that can make proactive quality a reality throughout the study lifecycle. It is also a powerful example of the value of descriptive reporting in a quality context, where the challenge is simply to understand the basic facts instead of to predict or diagnose. It helps fulfill an organization’s obligation to patients by increasing adherence to SOPs. But it also provides additional organizational benefit since study teams will already be carrying out these reporting duties in highly manual, inconsistent, and inefficient ways. Automating this work saves time and protects study budgets while ensuring consistency.
Case Study #2: Diagnosing Quality Event Trends
Reporting Quality Event (QE) trends is a foundational step for any quality organization. Since critical QEs require expedited handling to immediately control the issue, to protect study participants and data, to assess the impact, and to determine root causes and actions (including regulatory reporting), it is imperative to understand signals/trends to manage and control. A critical quality event typically refers to an unexpected occurrence or deviation in the manufacturing or distribution process that has the potential to impact the quality, safety, or efficacy of a pharmaceutical product.
The challenge is that simply knowing that there is a trend is never enough to manage it. Rather, what is needed is an understanding of the underlying causes and whether these causes point to an underlying problem. What’s needed is a move from the descriptive to the diagnostic.
Diagnosing the underlying causes for these trends can be problematic when an organization’s data cultures and systems are segregated. Commonly, QEs are tracked in an electronic Quality Management System (eQMS) that sits outside the scope of clinical operations and is therefore not natively connected to a host of data points which bear direct connection to QE trends.
A hypothetical example: Suppose (1) that an organization introduces a change which leads to increased site personnel turnover at various sites; and (2) that high volumes of QEs occur at affected sites after this change. All else being equal, this is precisely the type of relationship that is imperative for senior leaders to understand.
Crucially, factors like this can easily be missed when systems and cultures are not integrated. Because data points such as site personnel turnover rates are not (normally) housed within the eQMS, if the team responsible for diagnosing QE trends is limited to only the eQMS, then their reporting is inherently incomplete. Quality events occur in clinical and operational contexts, and it is a mistake to think that they can be understood in isolation from them.
One team faced this precise challenge: Reporting was not integrated and, as a result, the team struggled to move beyond the descriptive tier since it was unable to identify statistically significant relations between QE volumes and the various attributes housed solely within the eQMS. As in the previous case, the technical requirements for overcoming this challenge were relatively simple in outline. The team worked closely with data engineering and systems teams to integrate the operational and quality data domains in a centralized database, and to ensure their interoperability by linking study and site identifiers between the eQMS and Clinical Trial Management System (CTMS). This associated quality event records with the much richer set of properties housed in these applications, including study and site turnover rates, active patient volumes, consent withdrawal submissions, and other things.
This more comprehensive data set enabled the team to start testing hypotheses through deploying various statistical models (e.g., linear and logistic regression) and discover new statistically significant relationships.
Understanding the relationship of quality indicators to the fluctuations of a portfolio’s volume and mix, and understanding seasonal patterns in historical data, can help organizations begin to understand the broader operational context and its impact on quality. The diagnostic layer can also make it apparent which factors are impacted by quality. As such, the successful implementation of diagnostic analytics is one of the key milestones on any quality analytics roadmap.
Case Study #3: High-Risk Site Inspection Tool
The integration of systems and data, and the deployment of simple statistical techniques at the diagnostic layer, provide the foundation for predictive analytics: Now that we understand what and why things happen, we can begin to answer questions about what will happen in the future.
One team successfully deployed a predictive model as a tool for identifying sites at high risk for inspections. This tool built on the integration between the clinical operations and quality data domains to predict which sites within a study were at highest risk for being inspected by leveraging factors including number of randomized patients, number of protocol deviations, and previous inspections or audits (among many others).
Before deploying this tool, study teams were manually collating data on sites from across multiple systems, repositories, and manual trackers. This was highly inconsistent and relied on study team experience and intuition to move from the basic facts—e.g., the number of adverse events, protocol deviations, etc.—to a judgment in terms of the site’s risk profile. As a result, this case presented two opportunities to add value: First by replacing a manual process, and second by adding objectivity to the outputs. These two opportunities allowed for a phased approach which layered functionality and ease of end user adoption but also allowed for testing, validation, and training for each layer of functionality in the tool.
The first implementation phase was to take advantage of already completed foundational work that unified systems and data to develop a reporting solution which replaced the manual tracking processes. This solution not only captured existing functionality but extended it to visualize site information collated at country, study, project, and program levels. This approach benefited study teams by giving them a standardized approach while replacing manual data collation, reducing the potential for data-entry and data-quality errors.
The second phase was to embed a predictive model into the reporting solution to predict the risk of sites being inspected from 1 to 5 (1 being low risk and 5 being high risk). Happily, FDA and other regulatory bodies publish comprehensive documentation which explain their risk-based approach to the site-selection process. The team simply mirrored published methodologies as much as possible from the data available before validating the accuracy of the model based on standard statistical techniques. The model has proven highly effective at placing sites that had been identified for inspection into a risk score of 4 or 5.
Identifying tools and processes across your quality assurance, inspection, and audit portfolio that can uplift these capabilities one layer at a time can powerfully demonstrate the value of analytics approaches in the QMS space to the business. This roadmap should focus on quick, early wins to gain stakeholder traction and engagement on what analytics approaches can do for quality, hopefully leading to further engagement and investment from the organization in this space.