PicnicHealth
The time for change is now. AI can help bridge the gap between current inefficiencies in observational research and a healthcare system that learns from every patient’s experience. Our industry, unfortunately, has historically been risk-averse and slow to respond to disruptions. This time, we can’t wait. Sponsors that embrace AI will conduct research more efficiently and better match the right treatments to the right patients.
The False Dilemma between Off-the-Shelf Data and Site-Based Studies
Health data is siloed in many different systems. When patients visit multiple doctors and providers, tracking their health journey gets even harder. These scattered data often don’t get included in observational studies, making it difficult to collect the real-world insights the FDA wants.
Sponsors face a false dilemma when solving this challenge. They either buy existing real-world data or run a traditional site-based study. While off-the-shelf data can be quick and cheap to assemble from medical data or administrative claims data vendors, they may tell an incomplete or misleading story on their own. On the other hand, running observational research using traditional site-based methods may provide a more complete clinical picture, but these studies can be expensive and lengthy for sponsors and labor-intensive for research sites.
AI opens a better path to conducting observational studies more efficiently while also taking into account the complete patient experience. Sponsors can now bring together the best of both worlds: real-world data combined with direct patient connection in a virtual or hybrid study.
FDA recently published guidance explaining how to blend clinical trials into everyday medical practice. This allows researchers to gather data on approved drugs and well-studied drugs that doctors can safely manage in routine care settings. Moving forward with this type of approach, the industry can create a fluid, dynamic healthcare system that helps us better understand a drug’s safety and effectiveness and patient outcomes and, ultimately, bridge the gap between real-world realities and scientific rigor.
AI More Efficiently Reveals How Treatments Work in the Real World
At the recent HLTH 2024 Conference, FDA Commissioner Robert Califf highlighted AI’s rapid growth and how the regulatory agency needs to be responsive, yet flexible to new advancements, while continuing to improve patient health. Studying the real-world impact of treatments has guided the industry for decades. To unlock AI’s potential, while also transforming observational research, we should start by working directly with patients to tackle challenges in data interoperability. AI can, in turn, analyze millions of patient records across 100% of care sites in the US. This can replace slow, manual, laborious chart-review processes to quickly get insights on patient experiences.
AI is especially useful in finding insights in doctors’ unstructured narrative notes. One example based on a comparison of data from more than 600 lupus patients illustrates why this matters (see Figure 1). When looking at structured International Classification of Diseases (ICD) code data only, the prevalence of lupus nephritis, an often-unrecognized severe renal complication of lupus, appeared lower. But incorporating unstructured narrative text revealed that the disease was more common, improving the identification of lupus nephritis in RWD. With AI and other advanced technologies, we get a more accurate picture of real-world disease burden in less time than it would take humans alone to find these insights.
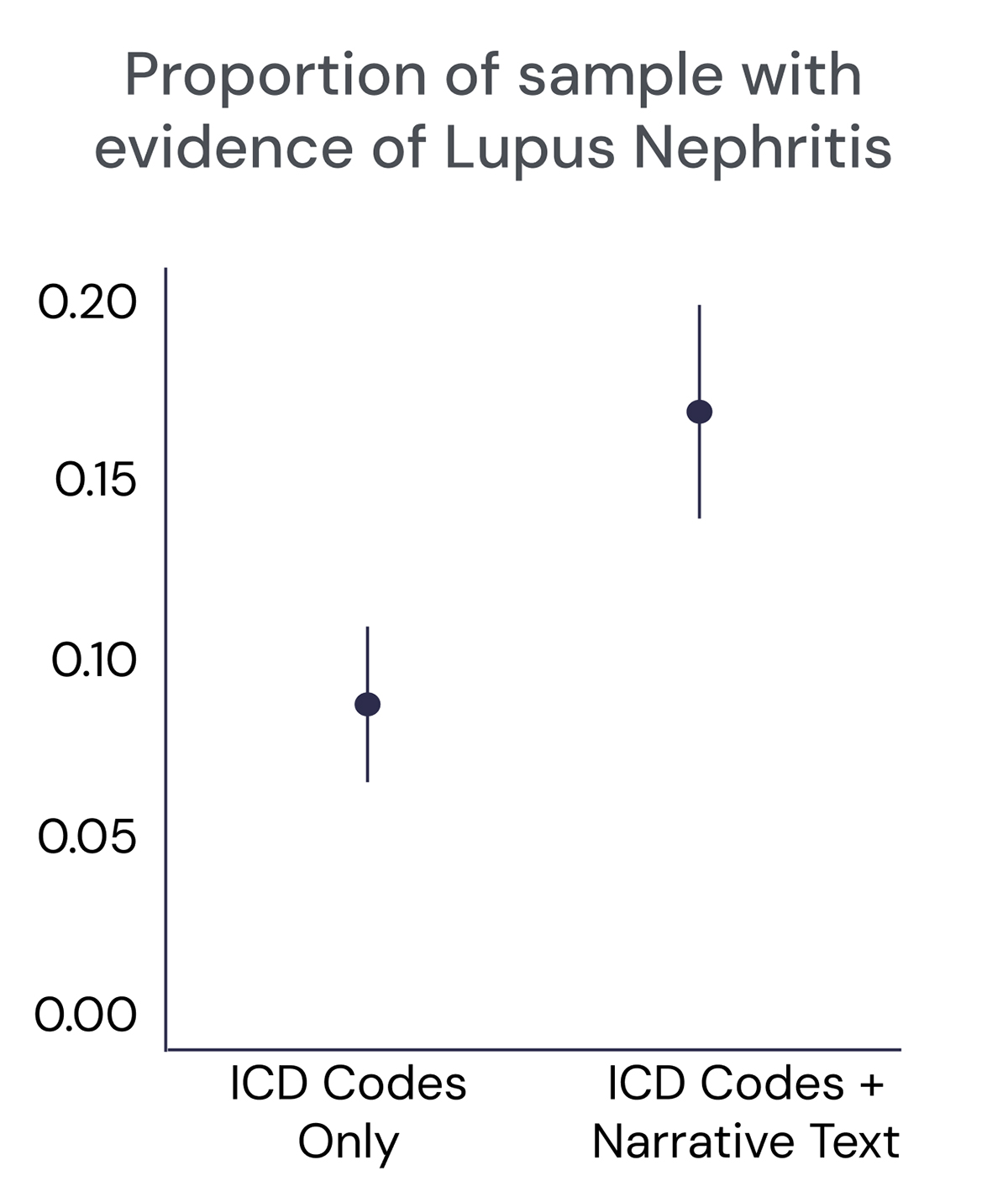
Match Treatments to Patients for Better Outcomes
Tracking drug effectiveness and safety using AI will help to drive improvements in patient outcomes and may decrease waste in the healthcare system. By understanding differences in how patients and their providers uniquely manage disease or utilize drugs based on a number of factors (such as age, race, and gender), we can uncover new precise and personalized ways that treatments can benefit more people.
Imagine the US healthcare system as a proactive, learning system that matches the right treatments to the right patients. Observational research can play a lead role. Studies can provide a faster, more comprehensive understanding of how diseases are diagnosed, progress, and are treated in the real world.
AI can enable a more fluid, dynamic system that learns from how providers document the more routine, day-to-day patient outcomes and behaviors to help make better decisions about which treatment options serve patients best.
For example, RWD has already highlighted the difference in healthcare resource utilization (HCRU) among women of different races with myasthenia gravis in the US (see Figure 2). Longitudinal medical record data captured across multiple provider types and care settings showed that nonwhite females had fewer total visits than white females but visited specialists more often. AI, with a human-in-the-loop, processed data (at scale) to generate the needed evidence.
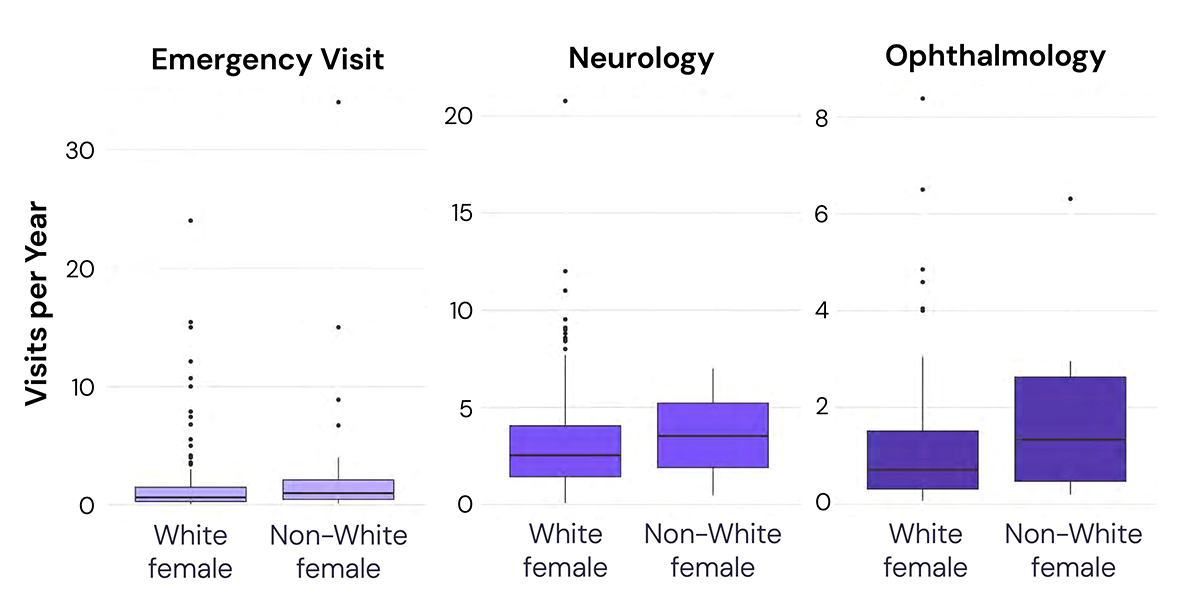
AI Powers a Continuously Learning Research Process
AI has rapidly advanced over the past two years since OpenAI introduced ChatGPT. There’s been rapid AI innovation and adoption since then, but the biopharma industry is still in the early stages of using it to understand the full patient experience. There is growing urgency to leverage advanced technologies to understand the increasingly complex patient journey.
The current siloed, reactive system is broken. We can now create a more proactive, learning healthcare system. Rather than relying on limited data or expensive interventional trial methods, AI enables a better path forward to find real-world insights.
Imagine a research ecosystem that is nimbler, patient-centric, and continuously learning. Rather than a siloed model, research can be deeply embedded into real-world care. Ultimately, this will lead to faster insights, more personalized treatments, and better outcomes for patients.