@indegeneinsight
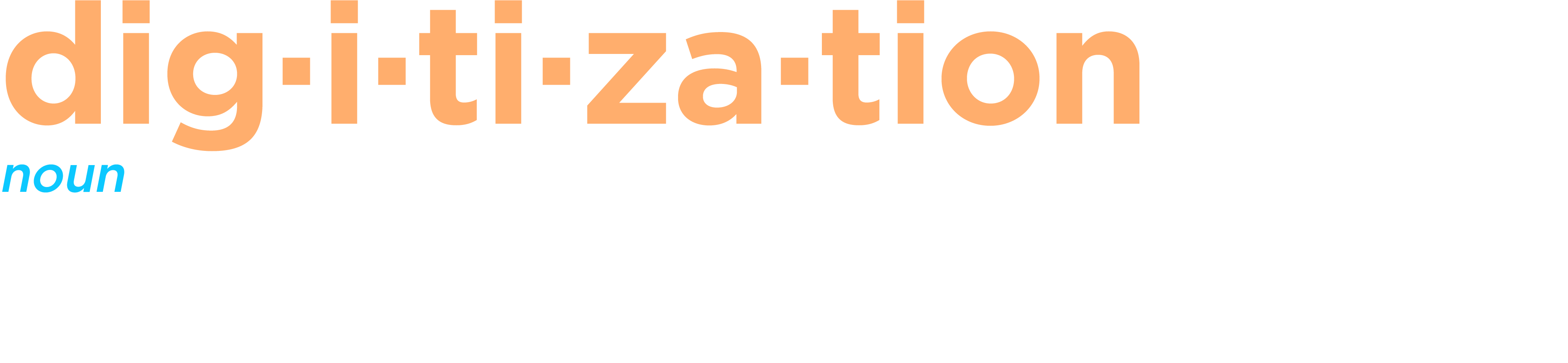
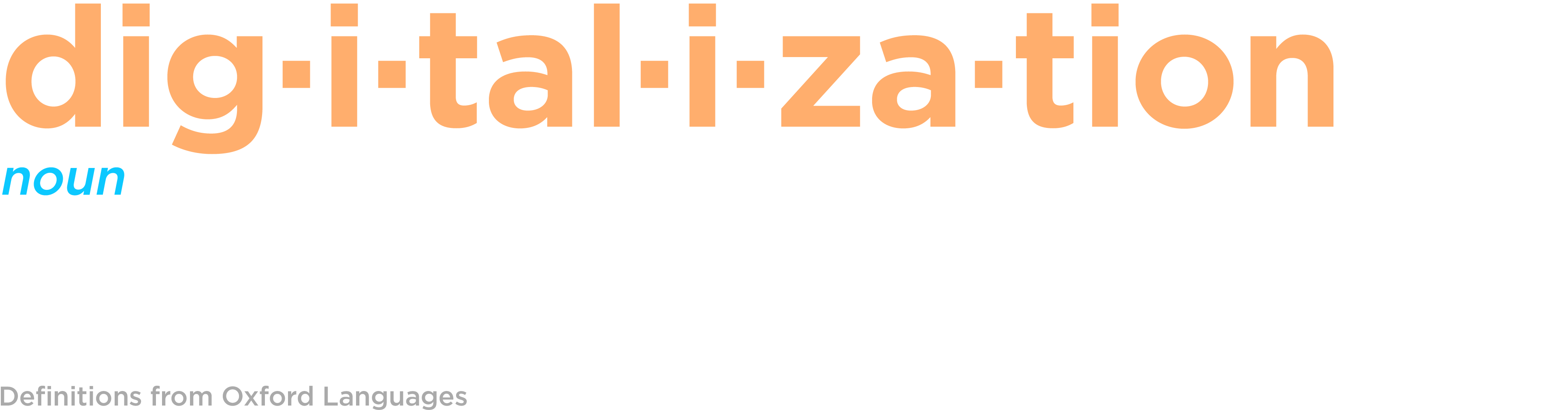
ecently, Generative artificial intelligence (AI) has emerged as a popular technology that has taken the world by storm. As a prime example, ChatGPT had already crossed 100 million users in January 2023, setting a record in just two months.
Foundational large language models such as GPT-4 (Generative Pre-trained Transformer 4, the latest version of ChatGPT released in March 2023 by Open AI) are significantly changing the way that domain subject matter expertise intersects with technology, with the healthcare and life sciences industry potentially becoming one of the largest beneficiaries. The impact that Generative AI technologies like ChatGPT could have on clinical, medical affairs, safety, and regulatory affairs functions may be profound. There are pitfalls to avoid, too. How can life sciences organizations leverage this technology to disrupt the industry for the better?
Generative AI can be used to create content in multiple forms, including audio, code, images, text, simulations, and videos. The technology could deliver targeted and customized information to specific stakeholders, ensuring swift decision-making. Features such as summarization might provide information that is both concise and precise. Generative AI could improve the productivity of skilled resources such as medical writers, address skill gaps, enable resource upskilling, convert data to intelligence, and deliver insights.
Here are some broad application areas:
- Classification and entity identification: Large language models such as GPT-3 and GPT-4 can identify and extract information from unstructured text and classify that information into fit-for-purpose domain taxonomies with a high degree of accuracy. This may well have broad applicability in domains such as safety, regulatory, labeling, and MLR (medical, legal, and regulatory affairs) review, as well as marketing and sales.
- Transforming content forms: While most news about these GPT models has focused on their capabilities using text and natural language, the new generation models are multimodal in nature. The ability of these models to interpret and generate not only text but images and other media is expanding. For scientific communication, this generative capability could greatly improve the efficiency of processes across life sciences’ medical and commercial teams. For example, clinical teams might benefit from table-to-text and text-to-table conversion while authoring clinical documents. Medical affairs and commercial teams may convert text to images and create a wide variety of infographics for medical training and promotional materials.
- Summarization and content translation: By chaining these generative models with embedding technology and vector databases, tasks related to summarization and synthesis of literature articles, large clinical/regulatory documents, etc., might be accelerated with new workflows that better capitalize on the human subject matter expert review. Content translation is critical to ensuring content availability across local affiliates. Generative AI solutions are likely to create an automated first draft of translated content and potentially improve the overall efficiency of content authoring across the entire drug lifecycle.
- Content analysis and synthetic data creation: It is possible to derive insights and strategies from large sets of data or content using Generative AI. Moreover, Generative AI models might also be used to cleanse data and create synthetic data to augment data sets while providing recommendations on the next-best action across various medical and sales-related interactions.
- Dialogue and response generation: Chatbots trained on a corpus of data might be created for a wide variety of use cases across training medical information, patient engagement, medical science liaison, and other professionals.
Mind the Gap and Be Aware of Pitfalls
- Accuracy: The outputs may not always be accurate or appropriate; they may also be biased and manipulated, or involve reputational and legal risks. Output from Generative AI can be indistinguishable from, or seem uncannily like, human-generated content. This is because the outputs from these models depend on the quality of the model and sources of truth used for training the machine to learn, the quality of training data, and the match between the model and the use case.
- Hallucination: Large GPT models have been shown to produce the “hallucination effect,” where the model creates facts or information that do not appear to be credible, accurate, or based on the user’s request. These artifacts may look convincing but have no basis in the real world.
- Data privacy and security concerns: There are challenges with the consistency, trust, and correctness of responses that Generative AI platforms create. The lack of clarity around data privacy, security, and compliance, as well as ownership and liability for such solutions, makes the adoption of Generative AI platforms in life sciences companies even more challenging.
- Garbage-in, garbage-out: The output is completely dependent on the quality of the query being asked. Akin to the questioner’s bias creeping into qualitative market research (which may lead to misleading responses), a whole field of “prompt engineering” is evolving to make sure comprehensive queries result in optimized and trusted responses.
As a result, although these Generative AI solutions are readily available, they might be difficult to use “as is” for healthcare and life sciences use cases where the critical point of care or regulatory decision-making is involved. These platforms must be augmented with current and accurate life sciences-specific data and operationalized using best practices with prompt engineering and human-in-the-loop processes.
Creating application wrappers (applications that leverage large language models but with an added secured layer around them) and fail-safe quality and trust mechanisms is also essential to ensure data privacy, security, and compliance. Finally, close integration between technology teams and business subject matter experts is necessary to comprehend current pitfalls and drive corrective actions to reduce “hallucinations” and improve output from Generative AI platforms. Life science companies are increasingly turning to partners with domain expertise and understanding of this technology.
So How Exactly Should You Use Generative AI?
Our team tested Generative AI applications across the medical value chain to identify key considerations through two case studies:
Example 1: Entity extraction out of free text, such as literature and patient narratives
The overall objective of this test was to enable precise entity extraction from free-text literature. The test involved “understanding the context” to separate adverse events from medical terms. The initial results did not show promise; however, resetting the right prompts yielded reasonably accurate results. In addition, leveraging further advanced prompts enabled the extraction of not only adverse events but the nature of the event, date, indication, medication start and end date, and other associated details.
Example 2: Leveraging a complex document for summarization
This test involved summarizing lengthy and complex medical documents, both text summarization and lay summaries. Initial results with open AI resulted in a “hallucination” which generated data that was not present in the article. However, after setting the right parameters in the generative model, including prompts and temperature (in this context, this refers to a parameter that controls the level of randomness and ensures the predictability of responses), the summarization and lay summaries were quite accurate—close to a human-generated output.
To ensure accurate and contextualized output from Generative AI, it’s important to focus on:
- Fine-tuning with validated, domain-specific data: Ensure that the right and contextualized data are used for model fine-tuning and prompt engineering. Avoid relying only on data that have already been used to train the models, because they may be outdated or lead to misinformation. Data used to train Generative AI systems should be representative, diverse, and not contain biases that the system may amplify.
- Validation and testing: Validate and test Generative AI systems using model performance monitoring to ensure their consistent safety, efficacy, and accuracy.
- Prompt engineering: Refine prompts or instructions passed on to Generative AI systems to trigger more accurate responses. In a fact-based domain like life sciences, engineering prompts to avoid biases and return factual instead of opinionated information is extremely critical, so establishing tools and processes to curate and manage the quality of prompts is important.
- Human in the loop: Review outcomes and improve the model fine-tuning and prompt engineering on a continuous basis.
- Focus on use cases: Avoid “hallucinations” by focusing on use cases where content is provided to Generative AI engines instead of asking them to fetch/create content.
Generative AI may well disrupt the life sciences industry, but human supervision remains the key.
Potentially, Generative AI presents an opportunity for the life sciences industry to get ahead of the curve and leapfrog other industries in adoption as well as success. Even so, being aware of the pitfalls and adopting best practices are essential to ensure safety, accuracy, and efficacy. If proper checks and balances are established, Generative AI may be instrumental in speeding up drug discovery by reducing costs and improving productivity across the industry.
The onus is on life sciences organizations to make optimal use of Generative AI for the betterment of global healthcare.