Special Section: Pharmacovigilance
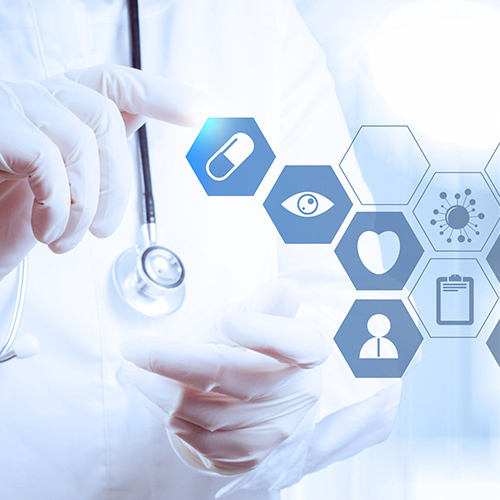
Austin Health, Melbourne
St. Vincent’s Hospital Sydney
or healthcare professionals in Australia, pharmacovigilance is a mix of centralized and decentralized processes. The pillar of these processes is the spontaneous report. In Australia, spontaneous reporting of adverse drug reactions (ADRs) may come from any source ranging from rural general practitioners, to urban hospital pharmacists, to patients themselves. Depending on the setting, these reports may go through thorough assessment and vetting (e.g., by a hospital ADR committee), or they may go through no such process. Heterogeneity is both a feature and a bug in this system. And this is a problem.
Artificial intelligence (AI) is often promised as a catch-all solution without any detail or thought, despite a sinking feeling that if it was, something would already have been done. What in practice stands in the way of applying AI to pharmacovigilance in a country like Australia, with its built-in advantages of universal healthcare and a largely single-payer system?
Collation of heterogeneous ADR reports in large databases like the Therapeutic Goods Administration (TGA) Database of Adverse Event Notifications (DAEN) is vital for signal detection in pharmacovigilance. This was first recognized in the wake of the thalidomide tragedy. Australian gynecologist William McBride first sounded the alarm about thalidomide in 1961, only after several years of widespread use. In its wake, stakeholders recognized that, with the right systems, future tragedies like this could be avoided. The TGA was established and, with the spontaneous reports as its pillar, the first era of pharmacovigilance began.
But if we are now facing an evolving landscape of therapeutics, what will the next era look like? How are we to tackle the problem of under-reporting, and the need for consistent reporting and rapid signal detection? We share the optimism about the power of AI in pharmacovigilance. But at the same time, the infrastructure that is required to empower this future is nascent and fractured.
Funding for healthcare in Australia may be centralized, but the organization and administration of healthcare are not. This has led to a fracture in the systems of healthcare data, including electronic medical records (EMRs) and prescription records. In 2012, the first iteration of My Health Record was developed to provide access to clinical data across systems, and although this system may help to bridge some of these gaps to facilitate patient care, no data linkage exists for signal detection. Systems like the Common Data Model (CDM) provide a way to link disparate clinical data sources. However, implementation of these systems can only facilitate data linkage; the linking itself still requires infrastructure, funding, and political will.
Data linkage is one of many issues that need broader courage and vision from all representatives from government, regulators, academia, industry, and healthcare beyond immediate expediency, to bring about reform and deliver the outcomes desired by all. In Australia, this currently relies on responsibility allocated to industry and driven by punishment rather than rewarding innovation and leadership, with reduced government investment now leaving expensive holes to fill later, and an absence of transparent reporting. It is no one’s specific fault, but such factors allow for avoidant, reactive behavior in which drug alerts are not made publicly available from a central location and therefore are not publicly available at all, instead of providing consistent and strategic multi-level clarity.
Even if all parties are aware of the problem and the benefits of certainty from a clear system, a revolution is unlikely to happen. But under the right conditions, deteriorating systems can drive inventive solutions and progressive change. Regulators have encouraged development, based on the knowledge that data science might provide economical and scalable solutions first to working around the system and then to eventually fixing it. ADR detection programs built in a single sub-jurisdiction or health system might eventually be the workhorse of drug safety in an integrated and universal manner; for example, academic-driven exercises can lead to usable insights and improved algorithms and overall reporting rates. These efforts are not wasted but are necessary evolutionary steps on the pathway to sustainable reform.
How can we encourage this? Systemic problems need systemic solutions, and innovation requires infrastructure that transcends current barriers. To actively contribute to a community solution, interested parties must have hope that their efforts will have broad impact as part of a logical plan. Administrative burdens keep data siloed, reducing the likelihood of scaling any given solution to the breadth of larger populations, and the consequent inertia discourages their further development. Even if the breadth can be traversed, the length of the process also contains many silos. The process currently segments into noncontiguous steps (identification, assessment of veracity, reporting, and assessing) each dependent on the other, but all with separate staff and data systems, and with information loss at each exchange. Veracity will naturally vary between assessments, but there is no chance to comparatively weigh sophisticated efforts differently than more basic lay efforts. Digital solutions could traverse these steps with the right governance and vision and provide the breadth, length, and depth to give sophisticated insights to increasingly complex problems.
Ultimately, in the near and foreseeable future, such systems would work toward an amalgam of the best of automated ADR detection with human integration, combining the best of both worlds to overcome each one’s frailties. We envision a future in which we will be able to identify ADR events using a sophisticated automated system based on natural language processing (NLP) over large electronic data sets from EMRs. In this scenario, ADR detection and assessment of veracity won’t be separate steps but will occur simultaneously, avoiding the data loss incurred through the less efficient steps of the current ADR determination process. Furthermore, once an event is identified, the probability that it is a true ADR is also determined and can inform the degree of certainty for each event.
To illustrate this point, consider myocarditis following receipt of a vaccine. An ADR report from a GP might detail chest pain after the vaccine. In contrast, in an automated scenario, all available information in the EMR would be considered: for example, a report of chest pain, a lab result that indicates elevated troponin, medical history showing that this is an elderly patient with prior CV risk, and triple vessel disease detected by angiogram. In this instance, a probability for the likelihood that the myocarditis is attributable to the vaccine will be determined and will inform further decisions.
The best people to build systems like these are those that understand the scenario and those that will use them. While numerous health professionals are involved across this process, in Australian hospitals pharmacists invariably take part and are often tasked with ADR identification and administrative processes. It stands to reason that pharmacists and automated ADR detection benefit from each other’s contributions. While not all pharmacists will become data science experts, their close involvement with implementation will contribute to a higher chance for success, especially in aiming for a national rollout. Their existing skills, such as “reading between the lines” in medical records to spot key information and communicating with clinicians, will undoubtedly help to successfully establish a shift to a new model, as well as in the interpretation of results. The heirs to the rich Australian pharmacovigilance tradition need the support of both artificial intelligence and clinical common sense.
Although ADR reporting is steadily deteriorating, we have the ability to build it back on new foundations. If recent advances in AI and computing are the tools, expert clinicians and pharmacists are the architects. The blueprint is ready.